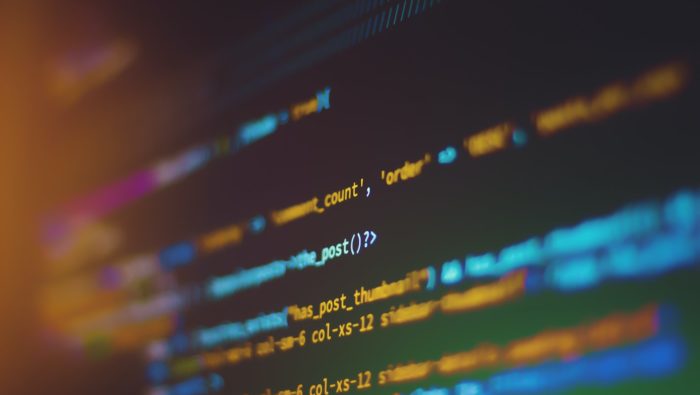
Global Security Infrastructures and AI: a Method Assemblage
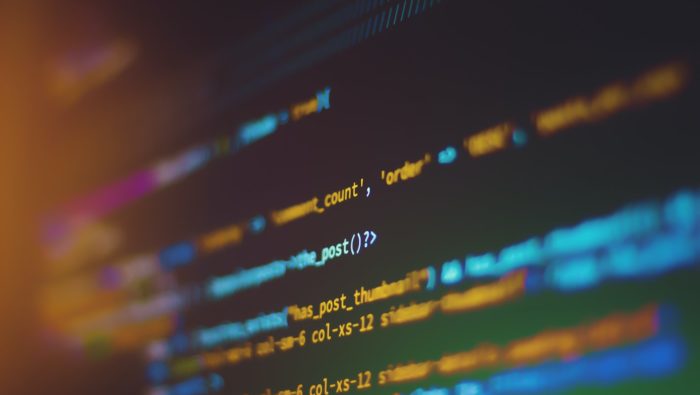
We are living in an era of pervasive datafication and planetary-scale computation, where global security risks are increasingly governed via predictive analytics made possible by rapid advances in AI. This intervention explores some of the methodological challenges of security datafication. Responding to Darian-Smith’s provocation to develop ‘global-socio-legal perspective[s]’ that can engage current transboundary problems, I briefly highlight two conceptual and methodological tools for doing empirical research in this space.
-
From data to infrastructures
When algorithms are framed as ‘black-boxed’ the key task becomes opening them up to make their inner workings intelligible. But counterterrorism data is sensitive and often withheld on security grounds. And even if the box were opened, it might not provide the answers we want. With advanced AI techniques ‘it will not always be possible for a human to fully assess the factors that the software took into account to form its conclusions’. Studying algorithms as situated practices within socio-technical assemblages or infrastructures is a more fruitful approach and something Socio-Legal scholars are well-equipped to do. As Gillespie argues, ‘we must not conceive of algorithms as abstract, technical achievements’ but ‘unpack the warm human and institutional choices that lie behind these cold mechanisms’. One suggested route is to follow how data is extracted, categorised and made ‘algorithm ready’. Another is to map the ‘classification dispositifs’ of data collection, cleaning and training practices through which ‘autonomous’ AI models do their knowledge work.
My current UKRI-funded research project – Infra-Legalities: Global Security Infrastructures, AI and International Law – develops this infrastructural approach for understanding how the movements of ‘risky’ people are governed. This includes analysing aviation data; processes for collecting and sharing it with Passenger Information Units; terrorist watchlist data; the global databases that circulate this information; AI-led processing to identify persons or behavioural patterns of interest; and the assumptions ingrained in algorithmic models and analysis about how ‘risky’ persons are constructed. Unlike the black box, data infrastructures are relational, built from interconnected local sites, inextricably tied to practice and capable of being empirically studied as socio-technical assemblages.
-
‘Following the data’: relationality and distributed agency
Widening the analytical lens this way has methodological and ontological implications. We come to understand algorithms through the socio-technical relations they produce and by following how they assemble actors, practices, knowledges, and artefacts in specific settings – what Bucher calls their ‘relational ontology’. This animates a different conception of agency as something distributed between human and non-human entities and alters our understanding of the law-technology relation.
When data moves between sites and scales or is reformatted for different uses it changes. My research on global listing has shown how seemingly mundane data interoperability initiatives that integrate different databases or make their data commensurable can generate powerful new reconfigurations of security law and governance in the shadows of ‘the law’. With the Security Council calling on states to ‘intensify and accelerate the exchange of operational information’ about suspected terrorists, and to collect and share biometric, travel and terrorist watchlist data, new opportunities for data-led security are proliferating. How, for example, do profiles built on patterns found in the algorithmic analysis of travel data bring new ‘risky’ subjects into being and enable new modes of governing global mobilities to emerge? Being attentive to such effects requires methods that can follow how non-human things (e.g., algorithms, lists, databases, classification practices) actively participate in the making of novel legal and political relations.
One way I engage this problem in my Infra-legalities research is to ‘follow the data’ of watchlisted people between sites and jurisdictions. Working with lawyers and NGOs, using FOIA requests and other disclosure strategies, we seek to map the security infrastructures that listed persons are entangled in and provide possibilities for them to challenge their targeting as ‘risky’. The aim is to understand how security governance changes as data moves between sites and as risk scores are calculated – creating knowledge to inform policy debates grounded in the dynamics of ‘algorithmic violence’ that puts the experiences of those most affected by AI-led security front centre of analysis.
Conclusion
Most legal scholarship regards algorithms as extra-legal and asks how ‘the law’ should respond to technological change. But this misses how law and governance are themselves being reconfigured through AI. Mapping the regulatory effects of data infrastructures and following how data and knowledge change as they move and interconnect are two methodological strategies for enhancing our understanding of the jurisgenerative effects of security datafication processes.